일 | 월 | 화 | 수 | 목 | 금 | 토 |
---|---|---|---|---|---|---|
1 | 2 | 3 | ||||
4 | 5 | 6 | 7 | 8 | 9 | 10 |
11 | 12 | 13 | 14 | 15 | 16 | 17 |
18 | 19 | 20 | 21 | 22 | 23 | 24 |
25 | 26 | 27 | 28 | 29 | 30 | 31 |
- 시계열 상관 분석
- Q-Q 플롯
- custom vision
- 순서형 데이터
- 군집화 시각화 방법
- 주성분 줄이기
- 계절성 모델
- R과 Python
- 선형 판별 분석 LDA
- 다중상관분석
- 데이터 종류에 따른 분석 방법
- Python
- 상위포지션
- 명목형 데이터
- 범주형 데이터
- ARMA 모델링
- 시계열 특성을 고려한 이상치 탐지
- Z-점수 기반 이상치 탐지
- 다변량 분석
- 시계열 모델링
- 상자 그림
- 주성분 분석
- ARIMA 모델링
- 데이터 분석 프로세스
- 지수평활법
- 최소-최대 정규화
- 데이터 수집 및 전처리
- 데이터의 차원 축소
- 상관 분석
- 날짜 시간 데이터 전처리
- Today
- Total
목록TIL/머신러닝 (9)
me made it !
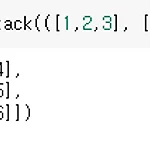
## 넘파이로 데이터 준비하기 fish_length = [25.4, 26.3, 26.5, 29.0, 29.0, 29.7, 29.7, 30.0, 30.0, 30.7, 31.0, 31.0, 31.5, 32.0, 32.0, 32.0, 33.0, 33.0, 33.5, 33.5, 34.0, 34.0, 34.5, 35.0, 35.0, 35.0, 35.0, 36.0, 36.0, 37.0, 38.5, 38.5, 39.5, 41.0, 41.0, 9.8, 10.5, 10.6, 11.0, 11.2, 11.3, 11.8, 11.8, 12.0, 12.2, 12.4, 13.0, 14.3, 15.0] fish_weight = [242.0, 290.0, 340.0, 363.0, 430.0, 450.0, 500.0, 390.0, 45..
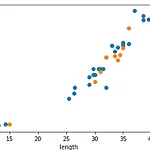
fish_length = [25.4, 26.3, 26.5, 29.0, 29.0, 29.7, 29.7, 30.0, 30.0, 30.7, 31.0, 31.0, 31.5, 32.0, 32.0, 32.0, 33.0, 33.0, 33.5, 33.5, 34.0, 34.0, 34.5, 35.0, 35.0, 35.0, 35.0, 36.0, 36.0, 37.0, 38.5, 38.5, 39.5, 41.0, 41.0, 9.8, 10.5, 10.6, 11.0, 11.2, 11.3, 11.8, 11.8, 12.0, 12.2, 12.4, 13.0, 14.3, 15.0] fish_weight = [242.0, 290.0, 340.0, 363.0, 430.0, 450.0, 500.0, 390.0, 450.0, 500.0, 475.0..
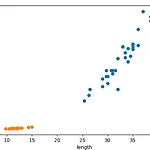
https://tensorflow.blog/install-python 윈도우즈에 아나콘다, 파이썬, 텐서플로 설치하기 텐서플로(TensorFlow)를 사용하려면 파이썬(Python) 개발 환경이 필요합니다. 파이썬 공식 사이트에서 인스톨파일을 다운받아 설치할 수 있지만 과학 계산을 위한 여러 파이썬 패키지를 따로 설치해 tensorflow.blog bream_length = [25.4, 26.3, 26.5, 29.0, 29.0, 29.7, 29.7, 30.0, 30.0, 30.7, 31.0, 31.0, 31.5, 32.0, 32.0, 32.0, 33.0, 33.0, 33.5, 33.5, 34.0, 34.0, 34.5, 35.0, 35.0, 35.0, 35.0, 36.0, 36.0, 37.0, 38.5, ..
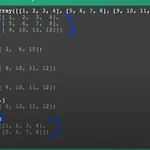
# Lab 4 Multi-variable linear regression import tensorflow as tf tf.set_random_seed(777) # for reproducibility x_data = [[73., 80., 75.], [93., 88., 93.], [89., 91., 90.], [96., 98., 100.], [73., 66., 70.]] y_data = [[152.], [185.], [180.], [196.], [142.]] # placeholders for a tensor that will be always fed. X = tf.placeholder(tf.float32, shape=[None, 3]) Y = tf.placeholder(tf.float32, shape=[No..
https://youtu.be/fZUV3xjoZSM?t=416 none : 값이 없다는 의미가 아니라 [현재 값을 정해주지 않는다 : 부정]는 의미 그 어떤 n의 값도 들어올 수 있음 # Lab 4 Multi-variable linear regression import tensorflow as tf tf.set_random_seed(777) # for reproducibility x1_data = [73., 93., 89., 96., 73.] x2_data = [80., 88., 91., 98., 66.] x3_data = [75., 93., 90., 100., 70.] y_data = [152., 185., 180., 196., 142.] # placeholders for a tensor that wi..
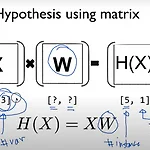
# Lab 3 Minimizing Cost # This is optional import tensorflow as tf # tf Graph Input X = [1, 2, 3] Y = [1, 2, 3] # Set wrong model weights W = tf.Variable(5.) # Linear model hypothesis = X * W # Manual gradient gradient = tf.reduce_mean((W * X - Y) * X) * 2 # cost/loss function cost = tf.reduce_mean(tf.square(hypothesis - Y)) # Minimize: Gradient Descent Optimizer optimizer = tf.train.GradientDes..
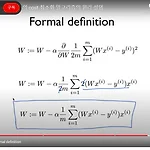
https://www.coursera.org/learn/machine-learning Supervised Machine Learning: Regression and Classification In the first course of the Machine Learning Specialization, you will: • Build machine learning models in Python using popular machine learning ... 무료로 등록하십시오. www.coursera.org https://holehouse.org/mlclass/ Machine Learning - complete course notes Stanford Machine Learning The following not..
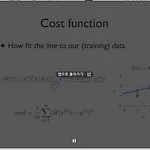
https://github.com/hunkim/DeepLearningZeroToAll/blob/master/lab-02-1-linear_regression.py GitHub - hunkim/DeepLearningZeroToAll: TensorFlow Basic Tutorial Labs TensorFlow Basic Tutorial Labs. Contribute to hunkim/DeepLearningZeroToAll development by creating an account on GitHub. github.com Linear Regression 선형회귀 분석 Cost function : 직접 세운 가설과 실제 데이터 값의 차이를 구하는 함수 # Lab 2 Linear Regression import ..
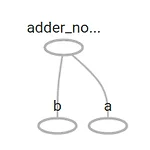
Getting Started With TensorFlow https://www.tensorflow.org/get_started/get_started Check TF version import tensorflow as tf tf.__version__ '1.0.0' Hello TensorFlow! # Create a constant op # This op is added as a node to the default graph hello = tf.constant("Hello, TensorFlow!") # start a TF session sess = tf.Session() # run the op and get result print(sess.run(hello)) b'Hello, TensorFlow!' Tens..